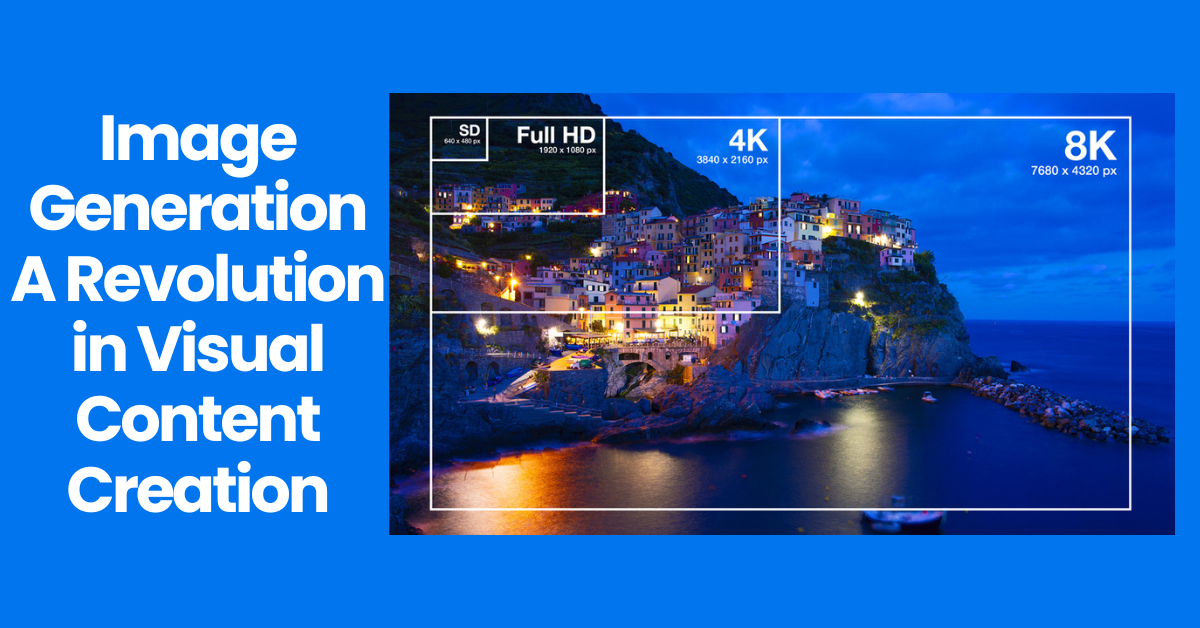
Image Generation A Revolution in Visual Content Creation
- Image Generators
- November 8, 2024
- No Comments
In recent years, image generation has emerged as one of the most groundbreaking advancements in artificial intelligence. This technology not only transforms how we perceive and create visual content but also reshapes industries ranging from art to marketing. As machines gain the ability to create strikingly realistic images from scratch or based on textual descriptions, the boundaries of creativity are being pushed further than ever before. This post will explore the myriad aspects of image generation, its underlying technologies, ethical considerations, and how it is changing our world.
The Rise of AI-Powered Image Generation: A Revolution in Visual Content Creation
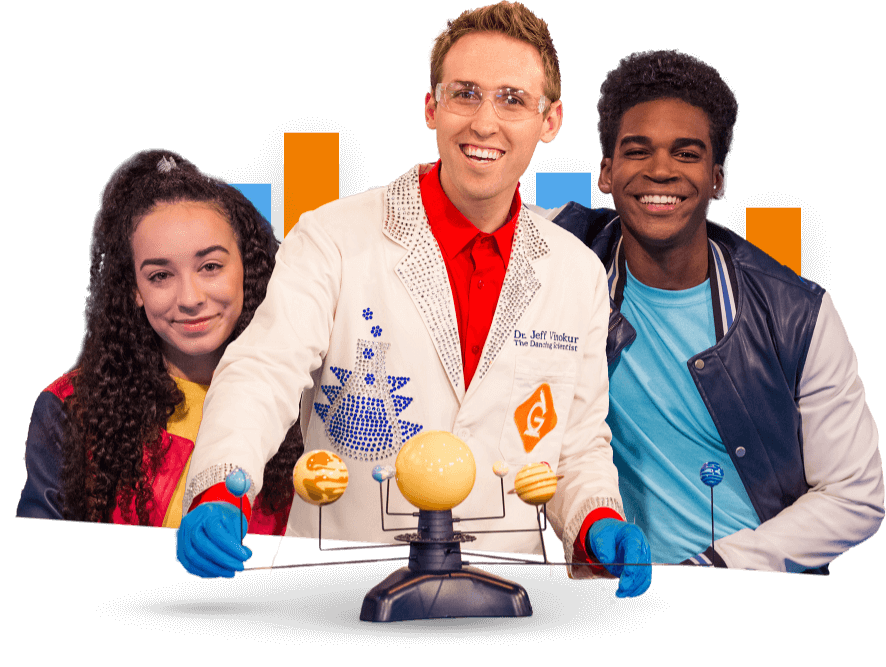
The advent of AI-powered image generation has marked a significant milestone in both technology and creative industries. With the power to produce high-quality images and visual content at an unprecedented speed, this technology is revolutionizing the way we think about creation and consumption.
The Transformation of Traditional Media
The rise of digital media has already altered how we consume information, but AI-driven image generation takes it a step further. Where traditional photography and illustrations were once the primary methods for visual storytelling, now algorithms can generate stunning visuals that can rival human artistry.
In this new landscape, artists and content creators have begun to integrate AI tools into their workflows, enhancing their capabilities rather than replacing them. For instance, graphic designers can employ text-to-image generators to quickly prototype concepts, while photographers can utilize AI to clean up images or even compose new shots that have never been captured. The fusion of human creativity and machine efficiency unlocks new realms of artistic exploration.
Accessibility and Democratization of Creativity
One of the most appealing aspects of AI image generation is that it makes creative tools accessible to everyone. You no longer need specialized skills or expensive software to create compelling visuals. With user-friendly platforms and cloud-based AI services, anyone with an internet connection can harness the power of image generation.
This democratization is particularly beneficial for marginalized communities whose voices often go unheard in conventional media. By providing easy access to powerful creative tools, AI image generation empowers individuals to share their stories, express their identities, and challenge stereotypes. In this way, technology becomes a bridge, allowing diverse perspectives to flourish in the visual landscape.
The Impact on Commercial Sectors
Beyond individual creativity, AI-generated images are making waves in commercial sectors as well. Companies are leveraging this technology for marketing, e-commerce, and social media strategy. For example, brands can quickly create tailored advertisements featuring products in various settings without the need for costly photo shoots.
Moreover, the use of AI-generated imagery can even enhance consumer engagement. Personalized visuals that resonate with target demographics can significantly improve brand loyalty and conversion rates. Consequently, businesses are increasingly investing in AI image generation tools to stay competitive in a fast-paced digital market.
From Pixels to Pictures: Exploring the Techniques Behind Image Generation
Delving into the world of image generation requires an understanding of the techniques that underpin it. These methods span a range of algorithms and approaches, each offering unique capabilities and applications.
Neural Networks: The Foundation of Image Generation
At the heart of most modern image generation techniques lies neural networks, specifically deep learning models designed to mimic human brain functioning. These networks enable computers to learn patterns in data, a process crucial for generating images that are coherent and visually appealing.
Neural networks consist of layers of interconnected nodes—much like neurons in the human brain—that process data inputs iteratively. When trained on vast datasets comprising thousands or millions of images, these networks become adept at recognizing and replicating features, styles, and textures found in real-world photos.
As such, when tasked with generating a new image, the network draws upon its learned knowledge to create a visual output that aligns closely with the input criteria. This capability allows for astonishing levels of detail and realism, transforming random noise into captivating images.
Generative Adversarial Networks (GANs)
One of the most revolutionary advancements in image generation is the introduction of Generative Adversarial Networks, or GANs. Proposed by Ian Goodfellow and his colleagues in 2014, GANs consist of two neural networks—a generator and a discriminator—that work in tandem to create and evaluate images.
- The Generator: This component creates images from random noise, attempting to produce outputs indistinguishable from real images.
- The Discriminator: This counterpart evaluates the generated images against real ones, providing feedback to improve the generator’s performance.
Through this adversarial process, both networks refine themselves over time, resulting in images of extraordinary quality. GANs have been used in various applications, from creating lifelike portraits to synthesizing entirely new environments for video games.
Variational Autoencoders (VAEs)
Another vital technique in image generation is the Variational Autoencoder (VAE). While GANs excel at producing high-fidelity images, VAEs specialize in understanding and manipulating the latent space of images.
Latent space refers to the hidden representation of data that captures essential features without all the noise. VAEs encode images into a compressed format, allowing for greater control over the generation process. Users can easily manipulate attributes such as color, style, and shape within this latent space, facilitating the design of customized visuals.
The strength of VAEs lies in their ability to balance reconstruction accuracy with diversity. They ensure that generated images are not only believable but also varied, enabling creative experimentation and artistic expression.
Democratizing Creativity: How Image Generation Tools Empower Everyone
One of the most exciting aspects of image generation is its potential to democratize creativity. By providing powerful tools to people from all walks of life, AI is breaking down barriers and fostering innovation across disciplines.
Low Barrier to Entry
Previously, creating high-quality images demanded extensive training and specialized software. However, thanks to advances in image generation technology, anyone with basic computer literacy can produce impressive visuals.
Platforms like DALL-E, Artbreeder, and DeepArt offer user-friendly interfaces that allow users to input text prompts or select parameters for their desired images. This simplicity encourages experimentation and creativity, making it possible for anyone to become a visual creator.
Notably, this accessibility fosters collaboration between artists, designers, and non-professionals alike. The blending of various perspectives leads to richer, more diverse creations that reflect a broader range of experiences and ideas.
Fostering New Artistic Movements
The rise of AI image generation tools is spawning novel artistic movements. Artists are incorporating AI as a collaborative partner, challenging traditional notions of authorship and creativity.
For instance, generative art combines human creativity with algorithmic processes, leading to innovative forms of expression that blur the line between human and machine-made art. As artists experiment with these tools, they often discover unfamiliar aesthetics and compositions that inspire further exploration.
Furthermore, AI-generated art installations are beginning to appear in galleries around the world, prompting discussions about the role of technology in contemporary art. These exhibitions not only showcase the capabilities of AI but also invite dialogue about the future trajectories of artistic practice.
Enabling Social Change Through Visual Storytelling
AI-powered image generation possesses enormous potential for social change. Individuals and organizations committed to advocacy can harness these tools to create compelling visuals that raise awareness about specific issues.
For example, non-profits can employ image generation technology to develop impactful campaigns addressing climate change, social justice, or mental health. By crafting engaging visuals that resonate emotionally with audiences, advocates can effectively convey complex messages and drive action.
Additionally, the ability to create imagery that reflects underrepresented communities empowers those groups to tell their own stories authentically. Such representations challenge harmful stereotypes and foster a more inclusive narrative in society.
Beyond the Hype: The Real-World Applications of Image Generation
While the concept of image generation may seem abstract or futuristic, its practical applications are rapidly becoming a reality across various domains.
Advertising and Marketing
In the commercial realm, AI image generation is revolutionizing advertising and marketing strategies. Brands can leverage this technology to swiftly produce personalized advertisements that cater to targeted demographics.
By analyzing user behavior and preferences, AI can generate tailored visuals that resonate with consumers. These customized ads are not only more effective but also reduce the time and financial resources typically associated with traditional campaign development.
Moreover, marketers can utilize AI-generated imagery for social media campaigns, ensuring a steady stream of fresh content that engages audiences. This adaptability allows brands to remain relevant in a constantly evolving landscape.
Fashion and Retail
The fashion industry is another sector witnessing the transformative impact of image generation. Designers can use AI tools to visualize collections before production, experimenting with colors, patterns, and styles in real-time.
Retailers benefit too, as AI-generated images allow them to create virtual clothing displays. Shoppers can see how different items look together, enhancing their online shopping experience. Notably, this reduces the need for physical samples and saves valuable resources in inventory management.
Film and Video Game Development
Image generation is playing an increasingly prominent role in film and video game development. Filmmakers can generate high-quality concept art, storyboards, and even entire scenes, expediting the pre-production process.
In gaming, AI-generated assets allow developers to create expansive worlds populated with unique characters and environments—all while reducing manual labor. This level of automation frees artists to focus on storytelling and gameplay mechanics, ultimately enhancing the user experience.
The Ethical Landscape of Image Generation: Concerns and Opportunities
As with any disruptive technology, the rise of image generation raises important ethical questions. Balancing innovation with responsible practices is crucial as we navigate this evolving landscape.
Intellectual Property Issues
A significant concern surrounding AI-generated imagery involves intellectual property rights. As machines learn from existing artworks to produce new images, questions arise regarding ownership and attribution.
For instance, if an AI generates a piece of art that closely resembles existing works, who holds the rights? Is it the programmer, the user, or the machine itself? These dilemmas necessitate thoughtful discourse among artists, policymakers, and legal experts to establish fair frameworks that respect creators’ rights.
Misuse of Technology
Another pressing issue is the potential misuse of image generation technology for deceptive purposes. With the ability to create hyper-realistic images, there is a risk of generating fake news, misinformation, or misleading visuals.
For example, manipulated images can be employed in political propaganda or social media campaigns, distorting public perception and undermining trust. As such, it is imperative to establish guidelines and safeguards that prevent the malicious use of AI-generated content while promoting transparency and ethical practices.
The Quest for Authenticity
AI-generated imagery challenges our understanding of authenticity and originality in art. As machines take on increasingly sophisticated roles in creative endeavors, the distinction between human-made and machine-generated art blurs.
This shift raises philosophical questions about the nature of creativity itself. Can a machine truly be considered an artist? Or do creative endeavors require human emotion and intention? Engaging in these dialogues will help us appreciate the value of both human and machine contributions to the creative landscape.
Generative Adversarial Networks (GANs): The Backbone of Image Generation
Generative Adversarial Networks (GANs) stand as a cornerstone in the field of AI image generation. Their unique architecture allows for remarkable capabilities in producing realistic images, making them a focal point of research and application.
The Mechanism Behind GANs
At the core of GANs lies an adversarial mechanism where two neural networks—a generator and a discriminator—work against each other.
- The Generator: Its objective is to create images that look convincingly real. It starts with random noise and refines its output through a series of iterations aimed at fooling the discriminator.
- The Discriminator: This network’s role is to differentiate between real images and those produced by the generator. It provides feedback to the generator on how well it is performing, shaping its learning process.
This iterative competition drives both networks towards improvement, resulting in increasingly sophisticated image generation capabilities. Over time, the generator learns to produce visuals that can easily pass as authentic, showcasing the immense potential of GANs.
Applications Across Industries
The versatility of GANs extends across various industries, demonstrating their ability to solve real-world problems.
In fashion, GANs can generate synthetic clothing designs, allowing designers to explore new styles and trends without the limitations of physical fabric.
In the medical field, GANs assist in generating training data for medical imaging, enhancing diagnostic algorithms and fostering advancements in healthcare solutions.
Additionally, GANs play a vital role in video game development, creating rich environments and character designs that captivate players.
Challenges and Future Research Directions
Despite their promise, GANs face several challenges. One major hurdle involves mode collapse, where the generator produces limited variations of images instead of a diverse array.
Ongoing research aims to address these limitations while improving stability during the training process. Moreover, exploring ways to increase interpretability and provide better insights into the decision-making of GANs is crucial for fostering transparency in AI-generated content.
Text-to-Image Synthesis: Turning Words Into Visual Realities
Text-to-image synthesis represents one of the most fascinating applications of image generation technology. This innovative process leverages natural language processing to create images based on written descriptions, transforming words into visual manifestations.
The Mechanics of Text-to-Image Generation
The process of text-to-image synthesis begins with encoding the input text description into a form that a neural network can understand. Natural Language Processing (NLP) techniques parse the input, extracting essential elements such as objects, actions, and attributes.
Once the text is encoded, the model utilizes various generative strategies, such as GANs, to produce an image that aligns with the written prompt. This complex interaction between language and visual representation enables machines to synthesize meaningful imagery from mere words.
Use Cases in Various Domains
The implications of text-to-image generation are far-reaching, touching various domains, including:
- Content Creation: Writers and bloggers can generate accompanying visuals that complement their narratives, enriching the overall storytelling experience.
- Education: Educators can create illustrations for instructional materials, making complex concepts more accessible and engaging for students.
- Gaming and Entertainment: Game designers can generate character models or environments based on narrative prompts, expediting the development process and enhancing creativity.
Challenges and Limitations
While text-to-image synthesis is a remarkable achievement, it is not without challenges. One major limitation involves accurately interpreting nuanced language and context. Ensuring that AI-generated images reflect intended meanings and subtlety remains a work in progress.
Maintaining consistency in style and coherence across multiple generated images is also an ongoing concern. Researchers continue to explore ways to enhance reliability and achieve results that resonate with users’ expectations.
The Future of Image Generation: Trends and Predictions
As we look to the future, the evolution of image generation promises to unveil exciting possibilities. Several trends are emerging that will shape the trajectory of this technology and its applications.
Increased Personalization
With advancements in machine learning algorithms, we can expect greater levels of personalization in image generation. AI will be able to learn more about individual preferences, tastes, and needs to generate visuals that resonate deeply with users.
This increased personalization will extend beyond marketing and advertising; it will influence creative works in art, literature, and beyond. Custom-tailored images will empower creators to engage audiences in unprecedented ways.
Integration with Augmented and Virtual Reality
The convergence of image generation with augmented reality (AR) and virtual reality (VR) technologies presents thrilling opportunities. Imagine stepping into a virtual environment where the scenery adapts in real-time based on your emotions or conversations, with AI-generated visuals dynamically responding to your interactions.
As AR and VR become more immersive, image generation will play a pivotal role in populating these environments with engaging and interactive visuals. This integration will redefine experiences across entertainment, education, and communication.
Collaborative Human-Machine Creation
The future of image generation will likely emphasize collaboration between humans and machines. Rather than viewing AI as a replacement for human artists, we will see more partnerships where machines serve as creative collaborators.
This evolution will shift our understanding of authorship, inviting diverse perspectives and encouraging interdisciplinary collaborations. As artists leverage AI to push creative boundaries, we can expect to witness groundbreaking works that blend human intuition with machine learning.
Image Generation in Different Industries: Transforming Design, Marketing, and More
The transformative power of image generation technology is resonating across various industries, leaving a profound impact on design, marketing, and many other fields.
Graphic Design and Prototyping
In graphic design, AI-powered image generation simplifies the prototyping process. Designers can create mood boards, mockups, and promotional materials in a fraction of the time it traditionally took.
By generating multiple design iterations quickly, graphic designers can focus on refining concepts rather than getting bogged down in repetitive tasks. This newfound efficiency enhances productivity while allowing for greater experimentation and innovation in design work.
Entertainment and Gaming
The entertainment industry is harnessing image generation to create stunning visuals for films, television, and video games. AI-generated content serves as a tool for animators and concept artists, providing them with the ability to visualize ideas before committing to detailed production work.
In video game development, AI-generated assets enable developers to expand game worlds rapidly, creating immersive experiences that captivate players. The combination of human creativity and machine-generated content opens doors for unique storytelling forms that engage audiences in novel ways.
Healthcare and Medical Imaging
In healthcare, image generation technology has remarkable potential for medical imaging applications. AI can assist radiologists in diagnosing conditions by generating synthetic training data that enhances the accuracy of machine learning algorithms.
Additionally, AI-generated visuals can aid in illustrating complex medical concepts for educational materials, improving patient comprehension and treatment outcomes. As the medical field continues to embrace technology, image generation will play a critical role in advancing healthcare solutions.
Building Your Own Image Generator: A Guide for Beginners
Creating your own image generator may seem daunting, but with the right resources and determination, it can be an achievable goal for beginners. Here’s a step-by-step guide to get you started.
Understanding the Basics
Before diving into building an image generator, it’s essential to understand the fundamentals of machine learning and neural networks. Familiarize yourself with terms like deep learning, GANs, and variational autoencoders.
There are plenty of resources available online, including tutorials, courses, and books, that cover these topics in depth. Take the time to explore and absorb this foundational knowledge, as it will be invaluable throughout your journey.
Choosing the Right Framework
Several machine learning frameworks are available to help you build your own image generator. Popular options include TensorFlow, PyTorch, and Keras. Each framework has its strengths and weaknesses, so consider your familiarity and comfort level when selecting one.
If you’re new to programming, you might want to start with Keras due to its simplified API, which allows for easier experimentation. Once you feel comfortable, you can explore more advanced frameworks like TensorFlow or PyTorch for added flexibility.
Gathering Data and Training Your Model
The success of your image generator hinges largely on the quality and quantity of your training dataset. Identify the type of images you want your model to generate and collect a diverse dataset that encompasses a wide range of examples.
Once your data is ready, preprocess it to ensure uniformity in size and format. Then, follow the guidelines provided by your chosen framework to train your model. Be prepared for this process to take time and require multiple iterations of fine-tuning.
Experimenting and Iterating
Creating an effective image generator is an iterative process. Don’t hesitate to experiment with different architectures, hyperparameters, and training techniques. Analyze the outputs and make adjustments accordingly to improve the quality and diversity of generated images.
Engage with online communities and forums dedicated to machine learning and image generation. Share your progress, seek feedback, and learn from others’ experiences. Collaboration enhances growth and provides valuable insights as you navigate the complexities of building your own model.
Conclusion
The realm of image generation is evolving at an astonishing pace, fueled by advancements in artificial intelligence and machine learning. As we’ve explored in this comprehensive overview, the implications of this technology extend far beyond simple image creation. From empowering individual creativity to transforming industries and sparking ethical debates, the rise of AI-powered image generation is indeed a revolution in visual content creation.
As we move forward, it is vital for stakeholders—artists, technologists, and policymakers—to engage in constructive dialogues about the opportunities and challenges that lie ahead. By collaboratively navigating this exciting frontier, we can ensure that image generation serves as a tool for positive change, inclusivity, and innovation in our increasingly visual world.
Looking to learn more? Dive into our related article for in-depth insights into the Best Tools For Image Generation. Plus, discover more in our latest blog post on Image Generator From Text. Keep exploring with us!
Related Tools:
Image Generation Tools
Video Generators
Productivity Tools
Design Generation Tools
Music Generation Tools